Using The Cloud To Connect Unstructured Data To AI And Analytics
By Russ Kennedy, Nasuni
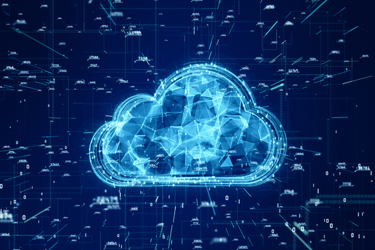
The desire to use analytics is no longer limited to the very largest organizations. In fact, one-third of business leaders surveyed by 451 Research said that business analytics was the top reason they were interested in using machine learning (ML). It’s no wonder. Big data analytics produce insights that can make an enormous and positive difference for business.
Architecture firms can analyze past proposals to uncover key elements that led to wins and losses, which can increase their percentage of successful bids. Online retailers can match customers with the products that they’re most likely to buy and then launch personalized marketing campaigns. Safety officers at mining companies can unearth indicators that predict when and where an accident is most likely to occur, prompting preventative action. And these examples barely scratch the surface of its power. Few technologies have been as transformative as ML and Big Data analytics.
However, to produce these kinds of results, ML and analytics need access to data and lots of it. That can be a big problem for many organizations because roughly 80% of enterprise data is unstructured (files, emails, images, etc.), which is typically stored in disconnected silos dispersed across many different locations. As a result, IT is rarely able to connect unstructured data with ML and analytics, which means that about two-thirds of enterprise data goes unused.
Certainly, it’s possible to connect unstructured, on-premises data to analytics, but for all but the largest companies, such an effort will likely prove far too expensive and cumbersome to undertake. IT can employ clusters and frameworks, such as Hadoop, to deploy analytics and AI on-premises, but it’s still going to be extremely challenging to feed terabytes of disparate data stores into the system. And even if this project is successful, if data storage locations are separated by long distances, performance will be challenging, because even the speed of light cannot overcome latency at big distances.
MSPs can help their clients overcome the challenges because if companies can migrate their data into the cloud, connecting data to ML and analytics is much easier, especially if the MSP partners with a hyper-scale cloud provider. AWS, Microsoft Azure, and Google all provide powerful AI and ML solutions within their clouds, such as Azure AI, Amazon EMR, Amazon Textract, and Google BigQuery ML. Even better, if MSPs provide their customers with cloud-based file storage solutions, they can unlock the power of ML and analytics for those organizations that would otherwise not have the means or resources to take advantage of them, especially for their unstructured data.
The Cloud Storage Connection To Analytics
It’s easy to connect cloud data to sophisticated cloud-based AI, ML, and advanced analytics services like those mentioned above that can crunch essentially any type of unstructured data an organization may have: images, text, video, CAD, etc. In fact, many of these services can be used by ordinary IT personnel. Most have simple point-and-click interfaces that don’t need a data scientist to obtain meaningful insights.
Additionally, the underlying object store architecture of major cloud providers is ideal for use with ML, AI, and analytics. Object stores are easily accessible, non-hierarchical, and extremely scalable, which is perfect for the massive data sets enterprises will want to feed into these services. They can access the data directly, without having to work through a complicated structure or tree. What’s more, object stores contain a wealth of associated metadata, which also can be analyzed to uncover even deeper insights.
Nevertheless, it’s still a challenge to move all an organization’s unstructured data into the cloud in the first place, because it’s not uncommon for some midsized enterprises to have multiple petabytes of information distributed across all their locations. For example, let’s say a company has a 1 GB/second upload connection, which is pretty fast. It would still take four months of non-stop transmission to transfer 10 PB of data. If the organization has a large amount of data and doesn’t have the luxury of a fast upload connection and lots of time, transferring data over the wire isn’t going to be a viable option.
Thankfully, that’s not the only option for getting data into the cloud. Strange as it may seem, organizations can have their data physically shipped much like a load of cantaloupes, and it’s faster than uploading it. Amazon Snowmobile, for example, will send a tractor trailer to the customer’s facility, transfer up to 100 PB of data to a rugged storage container and drive it to an AWS cloud data center. Remember, whatever method a company uses to get the data into the cloud, the process must be able to understand the original format to write it properly into the cloud’s object store.
However, it’s worth pointing out, if an organization is copying data to the cloud solely to connect that data to analytics, that’s a lot of additional management and cost without gaining any extra benefits. IT must now not only manage file data stored on-premises across multiple locations and systems, but also the copies of this data stored in the cloud, and it needs to be updated, backed up, encrypted, and secured.
One of the biggest advantages of transferring unstructured data to the cloud for AI, ML, and analytics, however, is that this type of analysis is far from the only benefit of storing enterprise information there. Once the data is in the cloud, a cloud storage service can replace traditional, on-premises network attached storage (NAS) arrays along with the backup and disaster recovery systems deployed to protect them.
Hybrid-Cloud File Services
For the last decade, cloud file storage services have grown in both their capabilities and customer adoption; most operate on a hybrid model. The master or “gold” copy of all file data resides in the cloud, where it can take advantage of its unparalleled resiliency, scale, and accessibility. To provide performance, the service caches the most frequently used files on a local appliance, with the deltas sent back to the cloud where they are then distributed back out to all other local caches so that everyone is working off the most current version. These services typically have some sort of file lock and automatically back data up in the background. If a file or entire fileshare is lost, corrupted, or infected with malware, all the admin needs to do is roll back to the previous copy. RPOs (recovery point objectives) and RTOs (recovery time objectives) can be measured in minutes. IT can redirect resources that were previously dedicated to file backup to other, more valuable projects.
Thanks to the cloud and the AI, ML, and analytics services they provide, these kinds of advanced data analysis technologies are now within reach of midsized enterprises. And now that cloud file storage services are mature and readily available, organizations can simply connect their cloud-based file share to cloud-based analytics services that don’t even require a data scientist. Honestly, there’s no longer any advantage to storing file data anywhere else but the cloud in this new age for IT.
About The Author
Russ Kennedy is chief product officer at Nasuni, which provides a file services platform built for the cloud. Before Nasuni, Kennedy directed product strategy at Cleversafe through its $1.3 billion acquisition by IBM. Earlier in his career, Russ served in a variety of product management and development roles, most notably at StorageTek (acquired by Sun Microsystems), where he brought several industry-leading products to market.
An avid cyclist and hiker, Kennedy resides in Boulder, Colorado with his family. He has a BS degree in Computer Science from Colorado State University and an MBA degree from the University of Colorado.