Is There ROI On AI For VARs And MSPs?
By Allan MacGowan, vice president of data analytics, CafeX
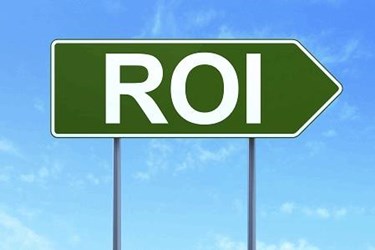
CafeX VP of Data Analytics Allan McGowan makes the financial case for "pragmatic AI" as a first opportunity for resellers, dealers, and integrators to leverage AI as part of their offering — especially in the contact center and telecom space.
According to a Forrester Research report, artificial intelligence (AI) is already transforming customer service by taking over predictable and highly repetitive tasks and enabling agents to focus on advanced interactions. The report states nearly 50 percent of consumers already engage in automated conversations with intelligent virtual assistants. Chatbots have become so commonplace many of us don’t event think about automated engagement as AI.
For most companies, it is necessary to validate whether the return on investment (ROI) is sufficient to justify the cost of deployment for AI-customer engagement solutions. The benefits of automation seem clear, but many customer support organizations still require detailed business case justifications before moving forward with a technology purchase.
Pure AI, in which machine learning and performance is indistinguishable from or even greater than that of humans, is apparently still years away. Until then, companies are turning to “pragmatic AI” which focuses on specific, commercially viable applications. This is represented by the rapid adoption of chatbots and other intelligent processes in customer service platforms which can typically handle simple queries that often do not require the expertise of a live agent. Such solutions will provide the first opportunities for resellers, dealers, and integrators to leverage AI as part of their offering, especially in the contact center and telecom space.
While quickly growing in capacity and capability, chatbots still only serve a slice of the larger omni-channel customer engagement spectrum. Substantial efficiencies in both operating costs and improved customer service metrics can be gained with chatbots. If an end-customer can quickly get what they need from a chatbot, it can save time and frustration. But when an engagement progresses beyond the capacity of the bot, it is critical for a business to offer a seamless escalation path to a live agent to deliver “the last mile” to customer satisfaction.
Placing a hard value on customer satisfaction is difficult, but a strong quantitative argument can be made for ROI strictly based on reductions in resources required to satisfy demand.
To assist resellers with sales efforts, we developed this ROI calculation method utilizing end-customer parameters to develop an AI-based contact center model that will produce an effective return in a reasonable timeframe. The calculator can be leveraged to convince business owners of the cost-effectiveness of chatbot technologies, helping dealers close sales and integrate AI capabilities into customer service infrastructures.
One approach to calculating ROI within a complex omni-channel customer service environment incorporates modeling live agent and automated agent capacities and performance alongside categorization of incoming query types. Using the case of one solution, CaféX Live Assist® for Microsoft Dynamics 365, this ROI model illustrates the inflection points where deployment begins to make sense. The general principles of the model can be applied to any chat solution, but without seamless integration across the omni-channel environment, the benefits may not be as substantial.
Step 1: Calculate Number Of Agents Needed To Handle Peak Time Interactions By Channel
The ROI model hinges on the ability of the customer service platform to redirect customer conversations from higher to lower cost channels. Let’s look at an example: In its current state, the support organization shown below predominantly interacts with customers via email, web portal, and voice calls. Using a combination of the Erlang-C algorithm plus industry standard values and customer supplied metrics, the model estimates the number of human agents currently needed (5,000) to process a specific number of conversations at a required service level during peak busy hour.
Step 2: Predict Number Of Interactions Initiated By An Intelligent Chatbot Vs. A Live Agent
The next step is to predict the future state after chatbot services are implemented. In this scenario, a percentage of voice, email, and web portal interactions are redirected to live chat conversations with chatbots acting as the initial point of contact. Using this example, no voice calls are redirected, but 15 percent of emails and 10 percent of portal engagements are front-ended by chatbots, with two out of every three automated interactions being escalated at some point to a live chat (and co-browse) agent. Calculating the new interaction volume by channel and re-running Erlang, the model estimates the number of live support agents is reduced from 5,000 to 4,390 — a reduction in agent headcount of approximately 12 percent.
Step 3: Compare Total Cost Of Ownership (TCO) Of Chatbots To Cost Savings Over Time
To translate this agent optimization into realistic labor savings, we estimate the total cost per full-time agent each year and take into account the fact that it may take months, even as much as a year, for the labor reduction to occur completely. Tangible economic benefits may not start accruing until the fourth month of deployment, reaching 100 percent only at the end of the first year.
To offset the benefits further, the model incorporates typical costs for implementation, including subscription licenses, professional services, a lab testing environment, and administrative staff to maintain the solution. Even with these conservative assumptions and all-inclusive costs, the benefits clearly outweigh the costs — payback on investment could be achieved well within a year. In the example below, the support organization achieves a 3X ROI during a three-year period, with payback occurring after only nine months.
The benefits will vary based on the characteristics of each contact center, the division of call types, the complexity of call topics, and the skills of the agents involved. In addition, there may be other hard costs associated with integrating chatbots into the workflow of some platforms. Without seamless handoffs for “the last mile” a company must anticipate some increase in soft costs associated with customer frustration and dissatisfaction.
One could argue the benefits can be even greater with the addition of co-browsing technologies. These innovations present attractive opportunities for capturing up-sell and cross-sell opportunities as agents visually guide visitors to relevant products at the point of purchase. However, with or without this additional functionality, Forrester is correct in stating that pragmatic AI is very compelling commercially and potentially transformative for customer service organizations.
Looking to the future of AI in customer service, chatbots serve as a strong example of a pragmatic, business-driven use case that provides an initial step towards much more sophisticated applications to enable efficiencies while maintaining customer satisfaction.
It’s unlikely that the future holds a completely AI-driven customer engagement ecosystem, but by providing these powerful tools, resellers can empower companies to offer specialized services when appropriate, while making the most of automated resources.
About CafeX
CafeX provides one-click collaboration solutions designed to help enterprises enhance team productivity and customer engagement within digital channels. Trusted by top global banks and other leading brands, CafeX empowers employees and guests to work together securely without friction from virtually anywhere using their preferred devices and tools.