Feed The Need: Preparing Customers For Big Data And AI
By Rich Itri, Eze Castle Integration
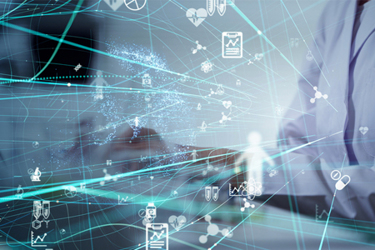
With digital transformation in full swing, the value of data is high enough to justify it being called “the new oil.” Further, it’s become the substance that fuels the engine of many businesses, driving key functions in industries from finance to manufacturing to retail. Today, data is being generated at record rates, not just across the IT enterprise, but increasingly from remote workers, cloud platforms, and the Internet of Things (IoT).
Yet, before companies can cull data and realize its benefits, first, it must be controlled – that means securing, storing, and creating a single source of the truth. Only then can you apply artificial intelligence (AI) and machine learning (ML) to filter fresh insights and identify new opportunities.
Two widely used methods for storage and analysis include data pipelines and data lakes. While managed service providers (MSPs) may be able to provide either, recommending the wrong approach can do worse than deliver watered-down results. In fact, it can set a company behind in its ability to compete.
Consider Your Options
A data lake offers a central place to store data at any volume. It can accommodate formatted, organized structured data, which is easy to search. It can hold unstructured data, too, though without a format, it can be difficult to collect and analyze. Data lakes are often referred to as the raw data layer because use hasn't been defined. Information in a data lake is not homogenized into the core dataset of a company (also is known as a data warehouse).
The key benefit of a data lake is that it can accommodate this greater diversity of data. In turn, users can more easily work with various types of analytics, including ML, while producing a higher quality of refined data. Organizations who implement data lakes can harvest details from other sources, too, such as social media and clickstreams.
A data pipeline is a framework typically made up of the following components: source data (such as third parties, SaaS, and IoT devices), destination (the abstract layer underlying storage), data workflow (business and data processes, validation, consolidation, monitoring), and the data storage layer (a data lake, data warehouse or blob storage).
Data pipelines are part of a system for filtering and formatting data. They're capable of delivering insights without excess information, the result being brief and easily reportable data. While they’re usually not used to analyze data, they can advance intelligence efficiency through customization aimed at addressing a specific business goal.
What’s Your Purpose?
The key to most effectively leveraging AI and ML relies on data consistency, quality, and depth of history. Pipelines help enable the flow of data into the data lake where the AI/ML tools can be applied. Data scientists refer to this process as "data wrangling."
Yet, while either option will work, data lakes and pipelines each offer distinct features and benefits, and by understanding how these fit into a company’s strategy, MSPs can effectively back into the data solution that will best match their customer’s needs.
For instance, ML requires large loads of data to unearth trends with significant potential. We often experience data lakes and ML without knowing when we use facial recognition on a mobile device. And, every time you use the feature, it takes in information and compares it with previous data sets to create a broader understanding. The more you use it, the more easily it will recognize you, even when you’re wearing an accessory like a hat.
Large data lakes can further benefit a business by allowing people to use their preferred analytic tools to sift data and find the information necessary for a specific purpose. This includes platforms like TensorFlow, which leverages an open-source library to help users develop and train ML models, and Jupyter, software created by an open-source project that supports interactive data science across all programming languages.
Data pipelines are the backbone of every AI-embedded app and largely the reason for their success. Why? If you had to unlock your iPad, and Face ID needed to scan every image detail in its memory, it would defeat the purpose; it would be a lot faster to key in a password. Whereas a data pipeline utilizes only relevant details that were accessed previously, the process is almost instantaneous. What's more, they get rid of conflicting and duplicate details to enhance speed even more, all while enabling companies to leverage disparate additional sources.
Simply put, pipelines can drive good data hygiene, master data management with a single source of truth, and improve overall stewardship.
Feed The Need
If a company requires a variety of data types from many sources – and they need flexibility for creative analytics – a data lake is the best bet. If they have a specific goal and need more precise details, then customization will be required. In that case, a data pipeline is the most effective way to process information down to the data lake, where it can be analyzed, propagated to a data warehouse, or enriched with other third-party data.
Note that one of the big challenges in the data space is maintaining and managing all the data pipelines needed to run an advanced analytics program. That's another area where an MSP can come into play. Those with the right framework and services can monitor, fix, and enhance data pipelines, keeping information accurate and up to date so analysts can focus on the output and not mundane operational tasks.
Data is the foundation of today’s digital world. AI and ML algorithms can help businesses identify and capitalize on opportunities, but these tools require volumes of data to truly deliver impactful results. Digital transformation is creating more and more useable information and it is clear Big Data is here to stay.
It’s up to MSPs to make sure their customers are ready, and those that can demonstrate this will win more prospects. After all, whether their approach will involve a data lake or data pipeline, if they do not jump in now, they’ll be left high and dry by competitors.
About The Author
Rich Itri is SVP of Professional Services at Eze Castle Integration.